Assimilation of remotely sensed soil moisture for an improved drought monitoring and forecasting
Despite the significant improvements in drought modeling over the last decade, accurate provisions of drought condition in a timely manner is still a major challenge. This study aims at operational drought monitoring and probabilistic forecasting over the Contiguous United States. The framework integrates statistical and dynamical modeling and issues drought forecasts at the lead times of 1, 2, and 3 months. The Variable Infiltration Capacity (VIC) hydrologic model is used across the CONUS and the associated uncertainties are quantified through Particle Filtering (PF) data assimilation by integrating observed satellite soil moisture with model estimates. The ensemble simulation characterizes the uncertainty in land initial condition and then a multivariate statistical model is used to generate probabilistic drought prediction. This framework is an enhancement of the NOAA’s seasonal drought outlook by offering drought probabilities instead of qualitative ordinal categories. Additionally, it provides the user with the probability maps associated in a particular drought category. The past drought event in the Great Plains and California were assessed and the results showed that forecasting of these costly droughts were possible at least one month in advance.
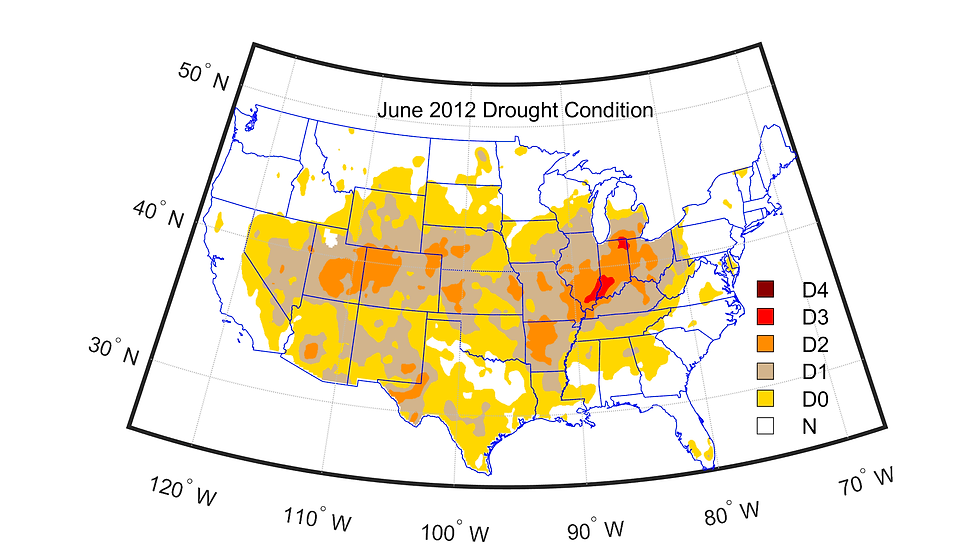
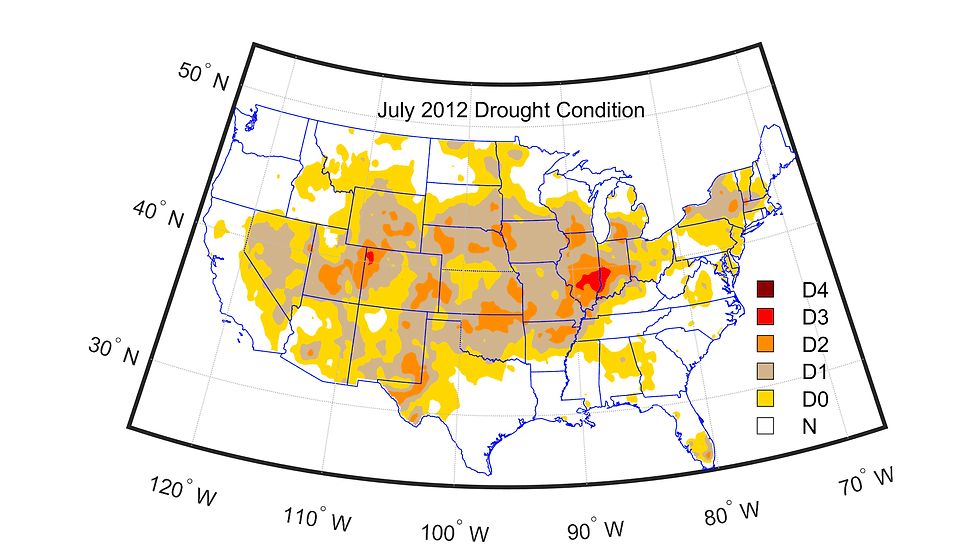
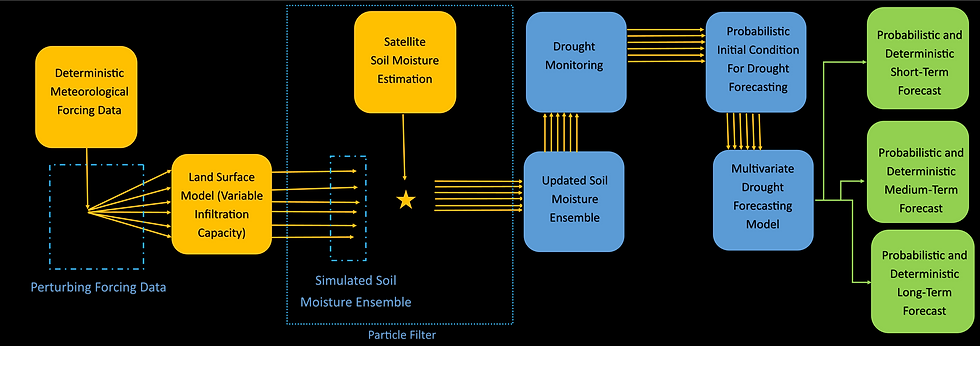
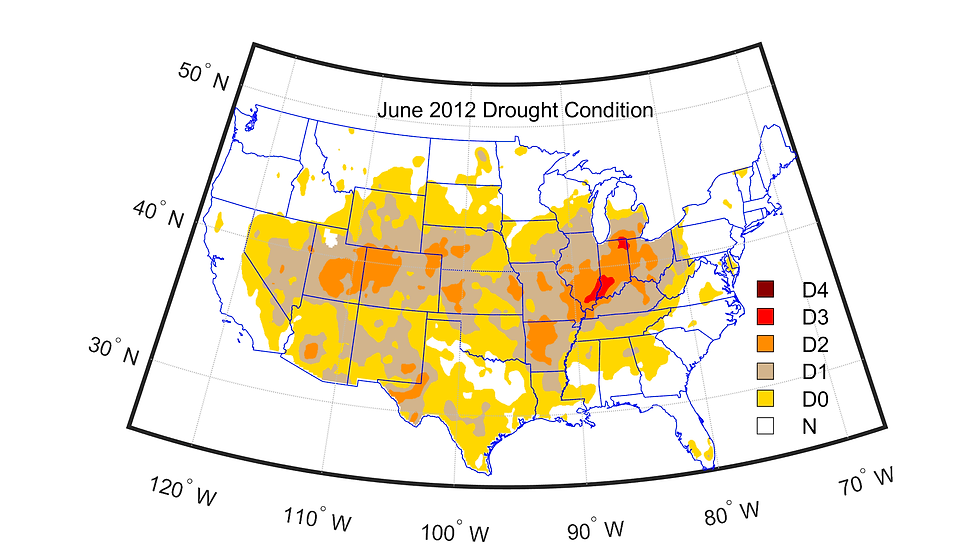
Operational Drought Monitoring and Forecasting (OPDMF)
The Operational Probabilistic Drought Forecasting System (OPDFS) is an online tool recently developed at Portland State University for operational agricultural drought forecasting over the Contiguous United States. This is an integrated statistical-dynamical framework issuing probabilistic drought forecasts monthly for the lead times of 1, 2, and 3 months. Operational drought forecasting is a challenging work and has been discussed numerously. A current available tool is the NOAA’s seasonal drought outlook (SDO) that issues monthly forecasts in form of four qualitative ordinal categories such as “drought persistence likely”or “drought removal likely”. The OPDFS builds on the NOAA’s SDO by offering drought probabilities instead of qualitative categories and provides the user with the probability maps associated with a particular drought category. The OPDFS offers a timely assistance to water managers, stakeholders and decision-makers to develop resilience against uncertain upcoming droughts.
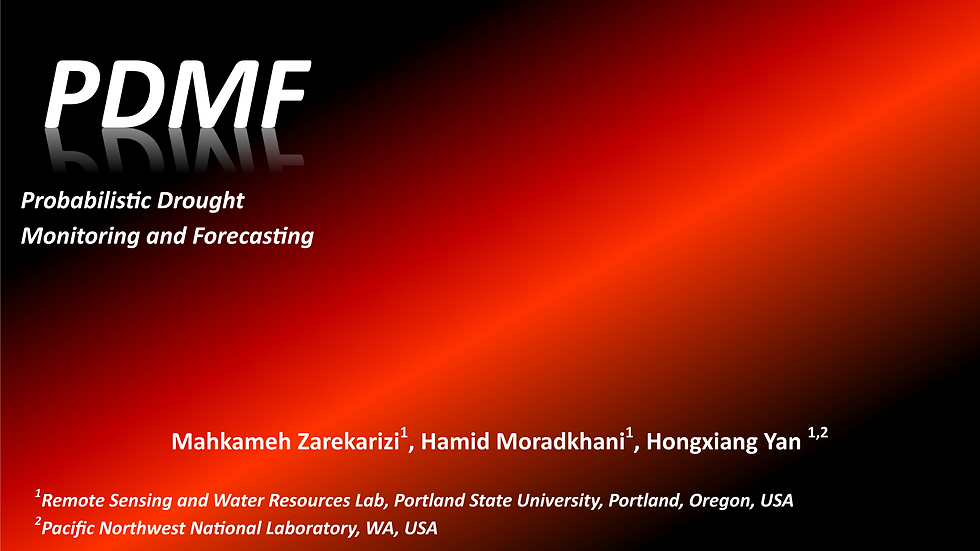
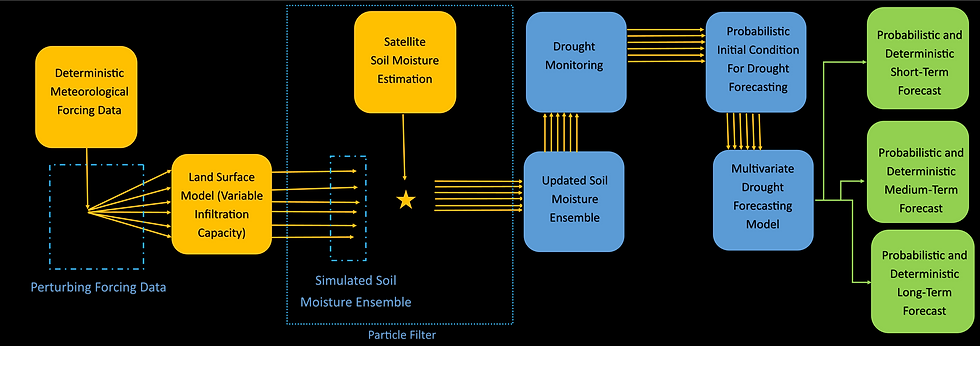

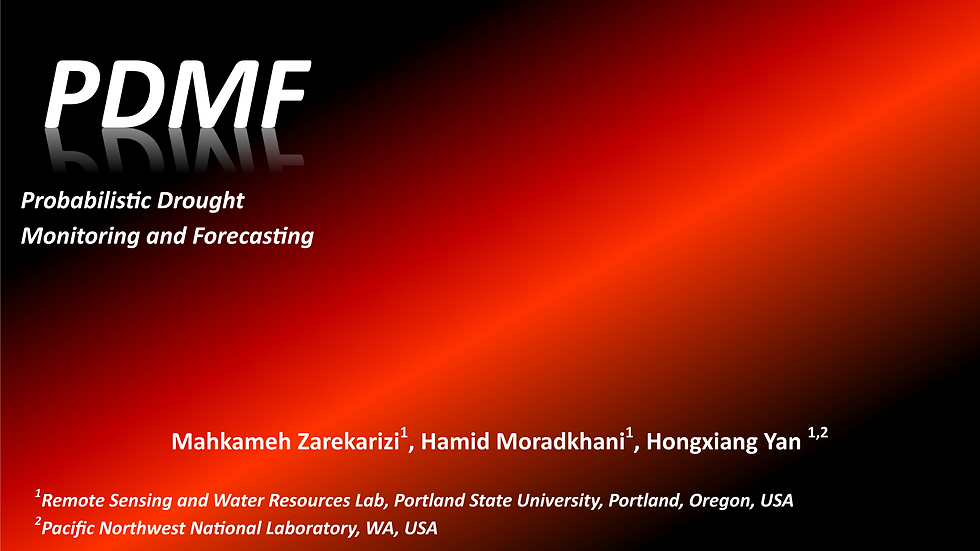
Projected Precipitation Extremes in the Pacific Northwest
Recently research has focused on the influence of climate indices on precipitation extremes. In the current study, we present the analysis of the precipitation-based extremes in Columbia River Basin (CRB) in the Pacific North-West USA. We first analyzed the precipitation-based extreme indices using statistically downscaled past and future climate projections from ten GCMs. Seven different precipitation-based indices that help inform about the flood duration/intensity are used in the study. These indices would give firsthand information on spatial and temporal scales for different service sectors including energy, agriculture, forestry etc. in the area. Temporally, historical and future projections are analyzed over the whole CRB using ten CMIP5 models. For each scenario, we have mapped out these indices over the area to see the spatial variation of past and future extremes. The analysis shows that high values of extreme indices are clustered in either western or southern parts of the basin while northern part of the basin is experiencing high increase in the indices in future scenarios. Here we focus our attention on evaluating the relation of these extreme and climate indices in historical period to understand which climate indices have more impact on extremes over CRB. Various climate indices are evaluated for their relationship using Principal Component Analysis (PCA) and Singular Value Decomposition (SVD). Results indicated that, out of fifteen climate indices used in the study, CRB is being most affected negatively by East Pacific (EP), Western Pacific Index (WP), Eastern Asia (EA) and North Atlantic Oscillation (NAO).
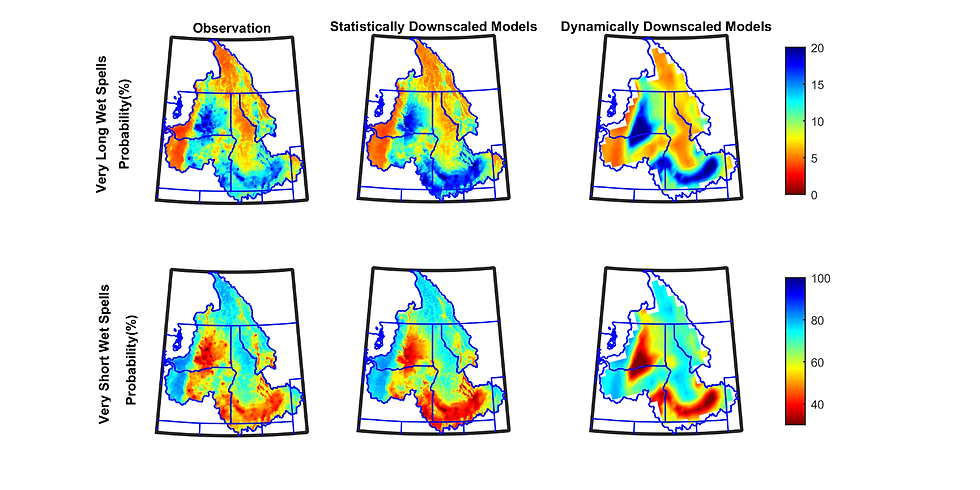
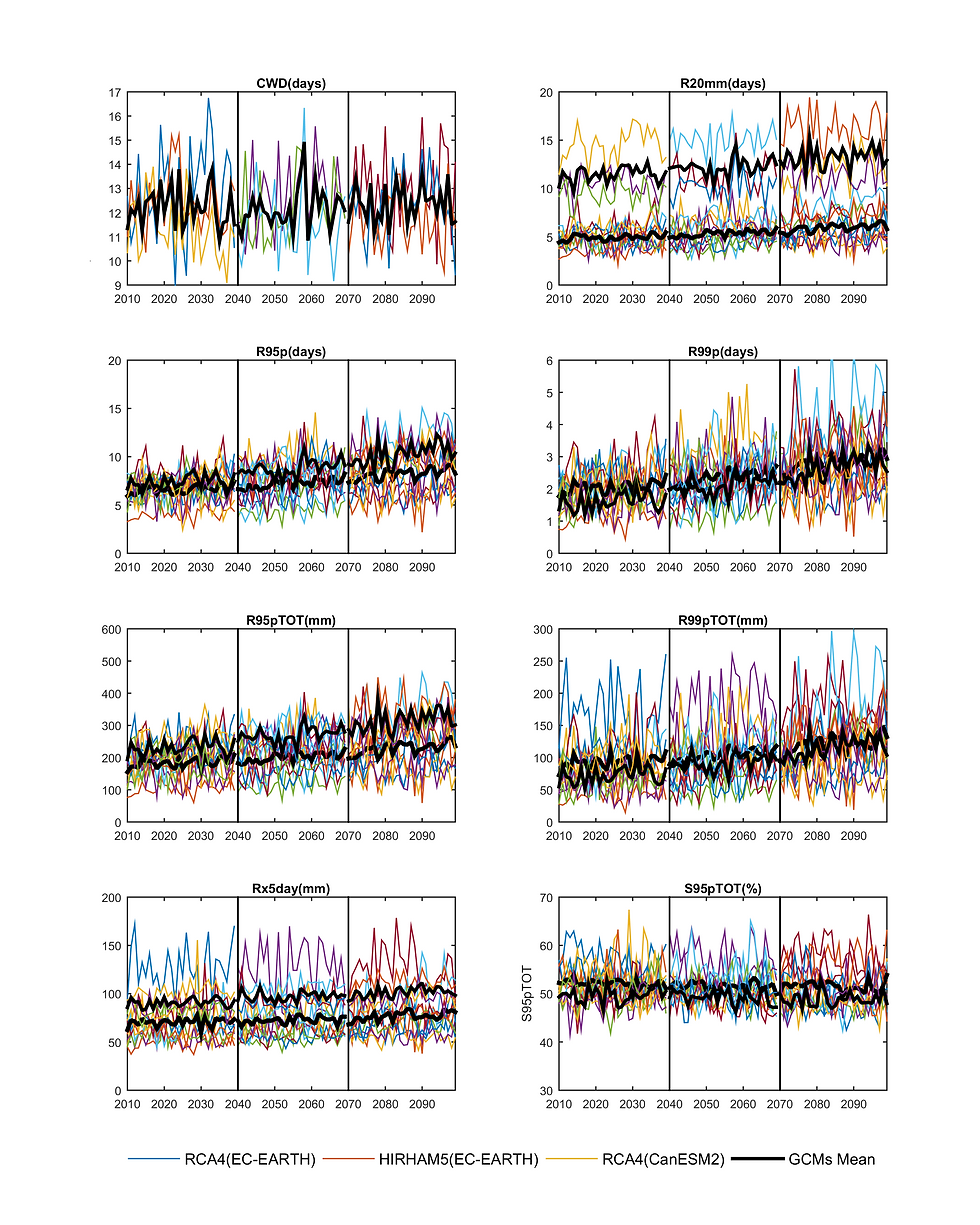

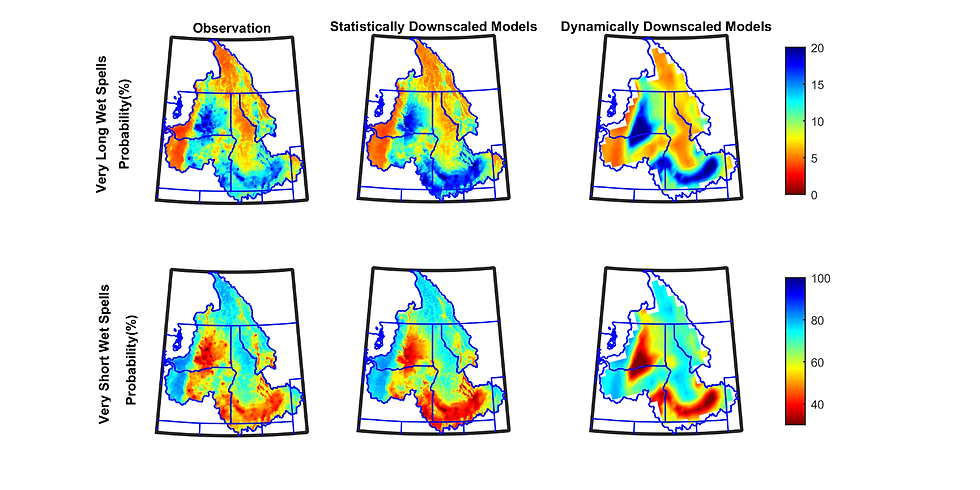
Spatio-temporal variations of drought recovery
Spatiotemporal variations of agricultural drought stages including onset, persistence, recovery, termination and retreat are analyzed probabilistically over the Contiguous United States (CONUS). Particularly, likelihoods of recovery from exceptional, extreme, severe, and moderate droughts at seasonal scales are evaluated. A multivariate statistical approach based on the bivariate Copula functions are applied to condition the soil moisture states on the previous season simulations[HM1] . Results show that the drought recovery likelihood is significantly dependent on the season and the geographical location. A notable conclusion is that the Western US has less chance to recover from droughts as compared to the Eastern US, implying two watersheds, on the two sides of the CONUS under similar droughts, respond distinctly with the eastern one being more probable to recover[HM2] . The results are verified by the US Drought Monitor and by the ability of model to detect the US historical droughts[AA3] . It was found that the drought retreat is more likely in spring than other seasons.


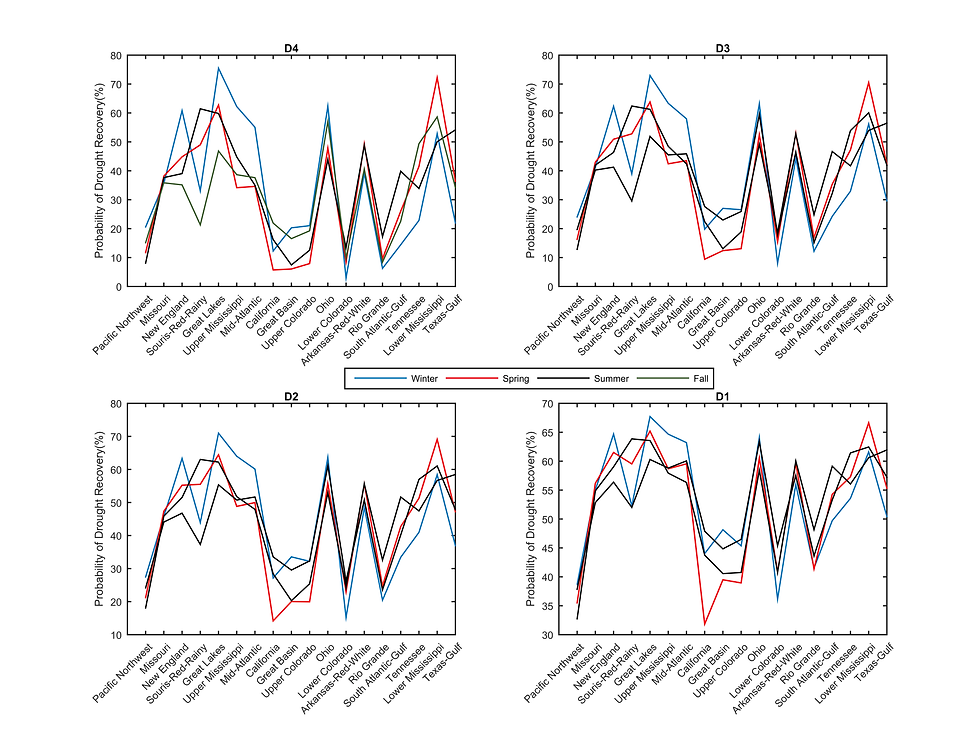

Non-stationary flood frequency analysis under climate change, climate variability, and urbanization
This study analyzes the linkages between floods and climate variables and utilizes this information for prediction of future floods. A framework is presented where the expected extreme flood for the coming year is predicted on early January using antecedent climate information. The framework uses a non-stationary hierarchical Bayesian model where model parameters vary in accordance with covariates. Cross-validation results show that the model has a high predictive skill. Comparative analysis between the stationary and non-stationary models reveals that considering non-stationarity improves the estimations considerably. In order to illustrate the effect of non-stationarity on practical engineering design, we also propose an approach for approximate estimates of non-stationary flood risk to simplify the complexities associated with the Bayesian modeling. Regression equations, curve plots, and scale factors are provided for approximate estimation of non-stationary flood hazards/quantiles. Johnson Creek watershed just upstream of Portland, Oregon in the Pacific Northwest U.S. is considered as the case study as it has demonstrated persistent flooding damages. Frequent floods in this area even after extensive flood control has become a concern for the city of Portland. We detected non-stationarity in the historical floods of this watershed, and found that the proposed approach can more reliably predict the extreme flood in this region.
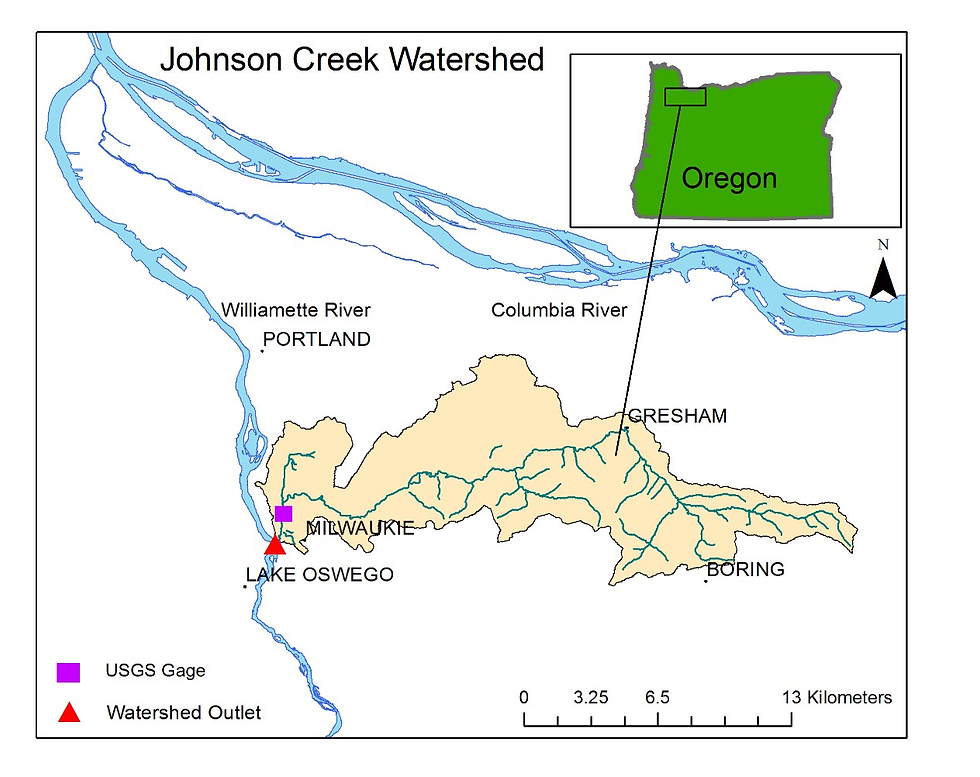

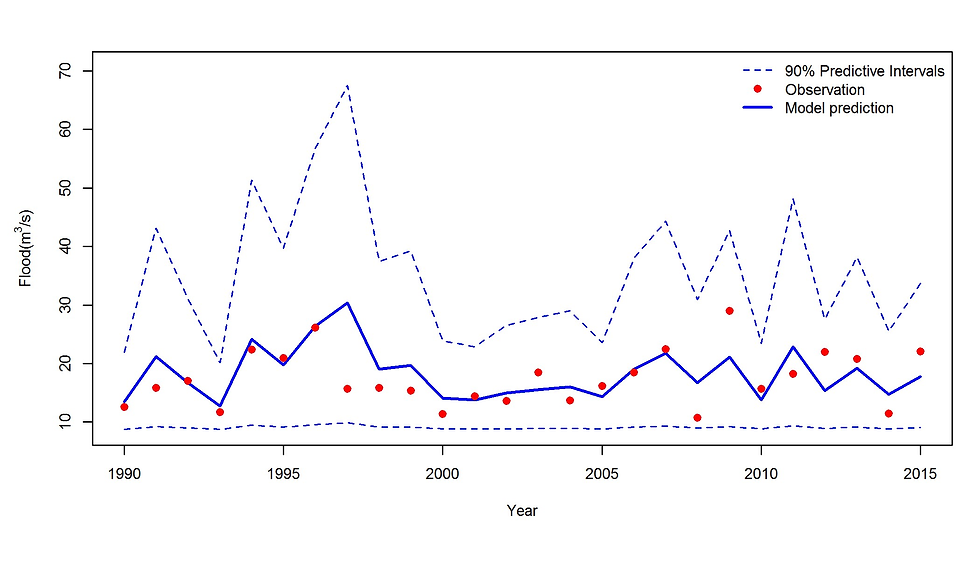
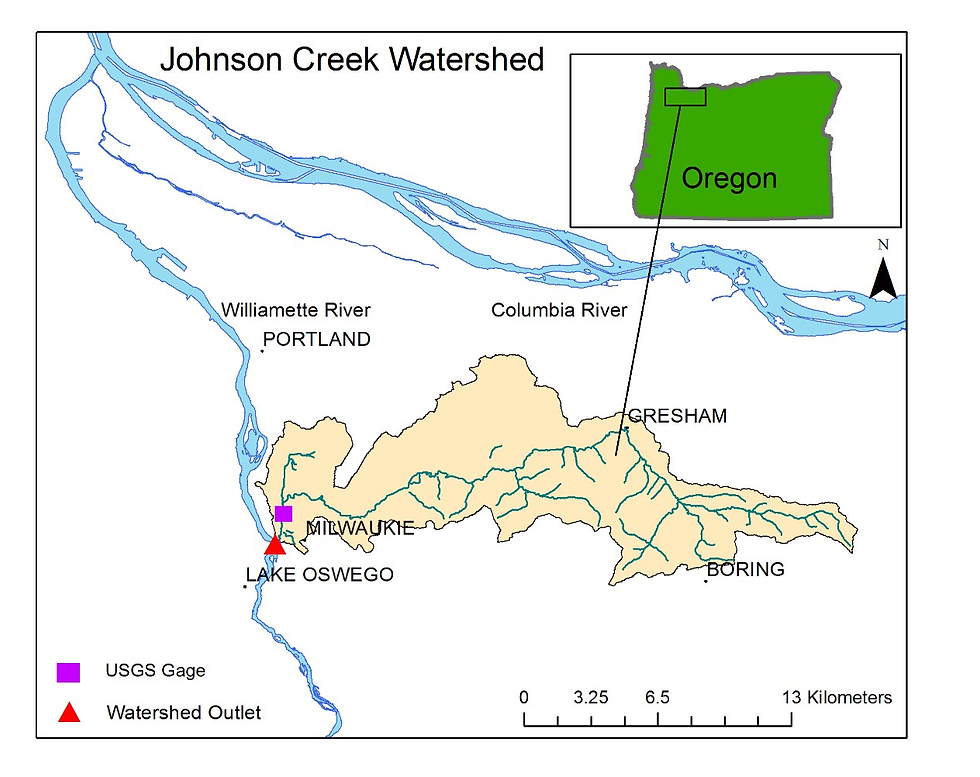
Currently: Multivariate data assimilation into the National Water Model for improved flood forecasts
Recently research has focused on the influence of climate indices on precipitation extremes. In the current study, we present the analysis of the precipitation-based extremes in Columbia River Basin (CRB) in the Pacific North-West USA. We first analyzed the precipitation-based extreme indices using statistically downscaled past and future climate projections from ten GCMs. Seven different precipitation-based indices that help inform about the flood duration/intensity are used in the study. These indices would give firsthand information on spatial and temporal scales for different service sectors including energy, agriculture, forestry etc. in the area. Temporally, historical and future projections are analyzed over the whole CRB using ten CMIP5 models. For each scenario, we have mapped out these indices over the area to see the spatial variation of past and future extremes. The analysis shows that high values of extreme indices are clustered in either western or southern parts of the basin while northern part of the basin is experiencing high increase in the indices in future scenarios. Here we focus our attention on evaluating the relation of these extreme and climate indices in historical period to understand which climate indices have more impact on extremes over CRB. Various climate indices are evaluated for their relationship using Principal Component Analysis (PCA) and Singular Value Decomposition (SVD). Results indicated that, out of fifteen climate indices used in the study, CRB is being most affected negatively by East Pacific (EP), Western Pacific Index (WP), Eastern Asia (EA) and North Atlantic Oscillation (NAO).
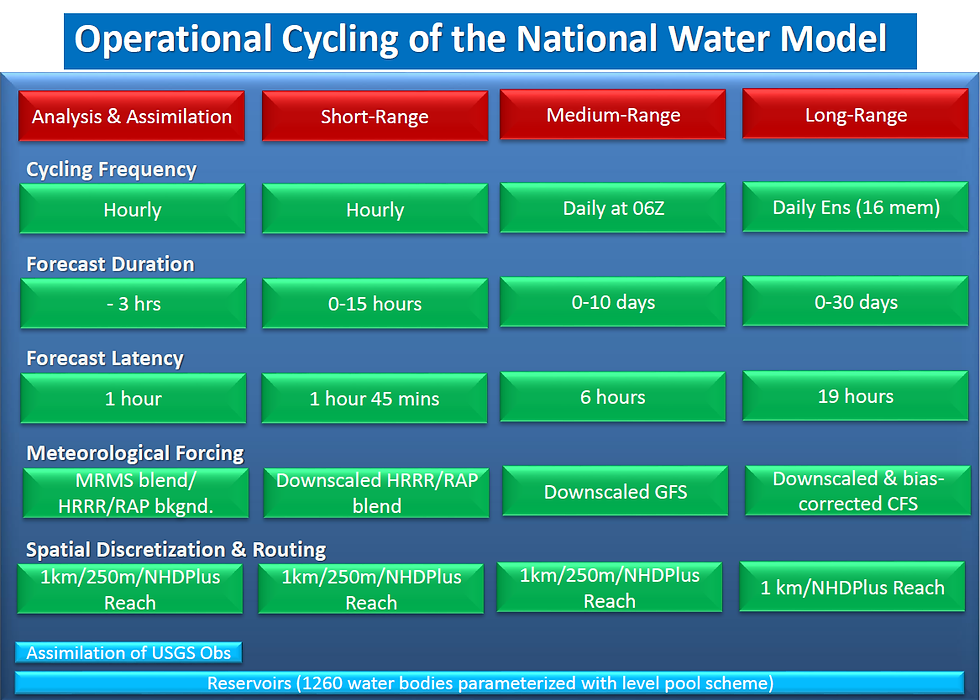

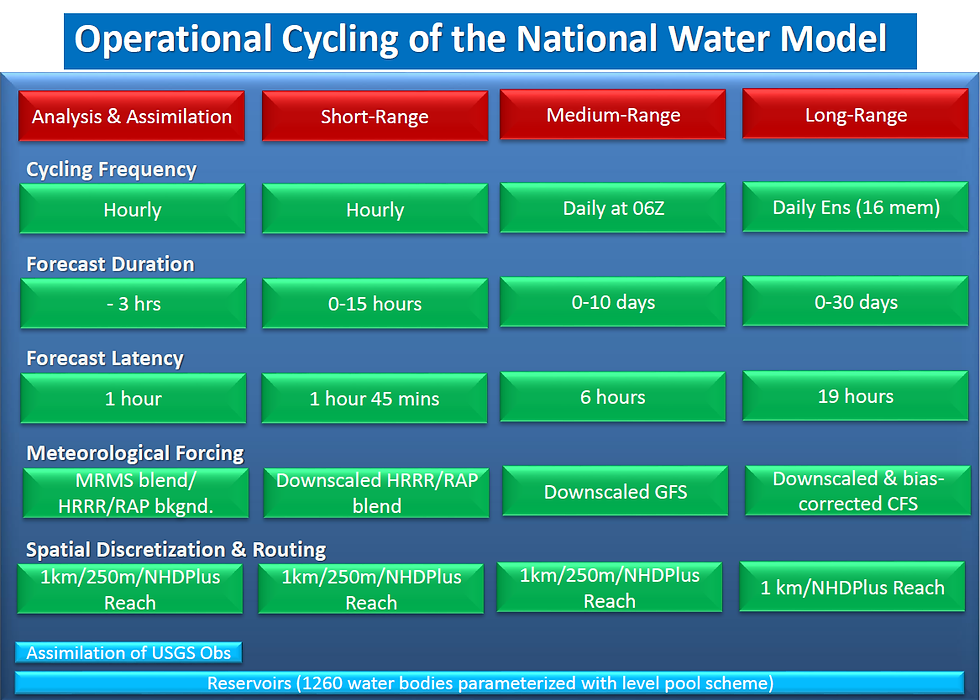